AI Adoption Starts with Product Management
- 0.5
- 1
- 1.25
- 1.5
- 1.75
- 2
Jon Prial: How long has it been since spreadsheets replaced paper, pen, and a calculator? A long time, right? That just seems like automation and productivity improvements are just another return of the crank, and the crank keeps turning. But guess what? There are still opportunities to bring technology to users of pen, and paper, and clipboards. Now we can do it to another degree. Why? AI. Have we got a podcast for you today. I'm John Prial, welcome to the Georgian Impact Podcast. With us today is Frank Emery, product manager at Fiix. Frank, we're glad to have you here. Why don't you tell us about what you do and who your typical customer is?
Frank Emery: Our typical customer at Fiix is companies with lots of assets. These can be as diverse as manufacturing companies that have giant stamping presses on big shop room floors, to schools, or gymnasiums that have lots of small assets, and they just need to be fixed. It really spreads across that spectrum, and across a lot of verticals too. So when we're building products for our teams, we're willing to think of things from that perspective. It has to fit a lot of different audiences, and a lot of different settings, dealing with a lot of different maintenance activities. My team in particular works on building AI tools to help make the older processes that these teams use more streamlined, and save them time. So we always say that we're looking for the scale problems and the complexity problems in traditional maintenance activities. We're looking to build AI to address those problems, and make them a lot easier for technicians to deal with.
Jon Prial: During the intro, I mention that your customers are not the typical office workers. They're getting to the next rev of Microsoft Office. I think the market is really interesting named CMMS, computerized maintenance management system. So I'd love to spend a little bit of time on the type of data that you get, and what your thoughts are on how you turn up the crank a bit more with the machine learning and AI, if you don't mind, please.
Frank Emery: For sure. I can say, and I always tell this to my team, we're very lucky in terms of data. I know a lot of AI companies out there have to source their data. But we don't have to. So a lot of the data we get is actually generated from our systems ourselves. So what happens is when maintenance technicians use the CMMS that Fiix builds, they enter in all of this great data around parts they're using, work they're completing, what broke, when did it break, how did it break? All of that gets stored in our data warehouse, in a structured, clean format. Our AI team actually gets access to all of that. So we can pull in that pre- structured data right into our machine learning engines directly, without having to do any additional work. Then the other set of data we get is actually IOT data coming off of different assets that customers might have that track how those assets get used, when they get used, if there has been problems, all that fun stuff that AIs love to eat up and use.
Jon Prial: When you think about gathering the data together, you've got lots of different companies, they may have similar data parts, whether it's different maintenance equipment, or on Windows, or whatever it might be. It is all your data, but the data does come from different company sources. How do you comfortably integrate them together and what's the message you have when you're talking to your customers about the value of that aggregated data?
Frank Emery: You know, that actually comes up a lot when we talk to customers. What I always tell people is when we ingest data from these different sources, what we do is everything comes in in its own data streams, and it's all encrypted, and secure end- to- end. I always tell people that we ingest it in aggregate, but we use everything separately. So a lot of our really large customers, for them, data security and trust is really important. So what we do is whenever we build AIs, or work with that data, we make sure that it's secured, and we actually build custom AI algorithms, and models, and training sets based off of that specific customer's data. So we kind of get the ability to do research on this big body of data. But when we actually build products for end customers, everything is specifically tuned to that single customer's instance, end- to- end, and there is actually no data blending, which people tend to find kind of surprising.
Jon Prial: Do you have shared learning from the data model? So for example, I love the fact that everyone's data is isolated, and they're getting custom algorithms against that data. But if you've got multiple school systems, for example, are there learnings from one school to improve your models, any sub markets, how do you begin to get value from being in more than one place in a similar space?
Frank Emery: So where we get value is really in the research side. So what we do is when we tend to build products, we do beta programs. What we'll do is we'll launch our AI models out to, say, 10 or 20 customers across a bunch of different verticals. What we pay attention to is the unique patterns in data, and how data is created and used. We build those learnings into our model generation algorithms. So a great example is the last beta we did, it was during COVID. We found that some of our customers had really interesting data patterns in how data was being generated in how parts of the work was being done. So what we did was we learned about those specific sub- patterns, and we built that learning into our model generation, so that other customers would get higher quality AI products, without having to actually share their data or have their data be used by anyone else. So all of this scale benefits that come from AI, we bake that into our research process.
Jon Prial: That is really awesome. So let's talk more about the users. Obviously these are maintenance people. They're not technical, they could be sitting on a shop floor. How do you work with them? Build a trust, and I'm thinking a couple of elements. I'm thinking of user interface, I'm thinking about messaging so they don't think you're taking their jobs away. How do you think about that? How do you keep your end users in mind?
Frank Emery: Well, for us it really starts with those beta programs. So whenever we're building something new, we always like to lead with that social proof. So a couple of customers that we have worked with really closely from start to finish, who can then talk about what we're building and how it's working for them, and how we're using data respectfully. Really to that end, when I'm picking beta customers, and we're walking them through the process that we're doing, I kind of come at it from two angles. I like to give them a high level overview of what specifically we're doing with their data. So I'll tell them, " Here is the exact data we're using. Here is what we're going to be doing to it. Here is the end result." I try to keep that understandable for them. So I try to work everything from the perspective that they're used to in the system. Then I go right to the value props afterwards. So what I was always taught was no one buys data. They buy what data does for them. So when we pitch these things, it's that emphasis on here is what I'm taking and doing with it, but more importantly, here is what you're going to get out of it. Then we have frequent check- ins. So for a lot of our beta customers, when we're building them something, I will talk to them every week, for the three, four, five weeks it takes to get through their beta program, making sure along the way that their concerns are heard, built into the product. Wherever there is concerns about data integrity, we address that right away. So with our current beta, we're doing a lot of really interesting work with maintenance requests, specifically. Out of the gate, customers said, " Please let us know how you're using our data to make sure it's being used respectfully." We jumped on that right away. We sent them all of our documentation, we jumped on the phone, we offered to have them talk to our machine learning team as well, tom ake sure that they knew that everything that we are pulling out of the system was being pulled out securely.
Jon Prial: Yeah, you reinforced for me why I think product management is the most interesting job in all of software. So as you're working through these betas, and you're constantly talking to your customers, I think that's fantastic, and you're listening to what they say, it's evolving realtime. You're hearing what they're saying in realtime, and then turning it back again in terms of as the product evolves, and as your messaging evolves. So tell me more about the iterations you do with your customers.
Frank Emery: Yeah, absolutely. That's one of the reasons we've had so much success so far, with their AI products, is because we have that fast iteration. It usually breaks into two pieces. So when we start beta programs and we start talking to customers, the first thing we always like to validate is, is our model spitting out the right thing? If your model isn't spitting out the right results, there is no point in really focusing on anything else, because you might have to figure out a new business process or a new end product that you can rebuild in. So we start the conversations by saying, " Was this prediction accurate? Yes or no?" Then usually those conversations turn into a discovery process about that particular user's businesses or maintenance processes that help us build and reinforce models later on. So when we did our first parse prediction model, the end AI that we used and put into production was probably not in any way what we first developed, and it's because of that feedback that we got along the way from talking to customers, and building in how specifically they run their business. Once the model's predictions are coming out the way we want, it gets into the UI phase of things. Which in a lot of ways, can be kind of trickier, because our user base isn't as technical as you might be used to. So a lot of times, when you think of AI, you probably think of a very technical team using it. But we're building AI for people who really want nothing to do with computers. They want an end result or an answer. So when we get into the UI development phase of things, it becomes a discussion of, " Here is the result, does it make sense to you? Is it clear what you have to do next? Is this something you think is going to be really useful?" The trick is because we're deploying these new AI insights into these pre- existing processes, the rule of the game is it has to integrate with their existing processes in as seamless a way as possible. That's where the UI work comes in, is how do you get these predictions and these insights from the AI fitting into these user's processes and existing workflows? Again, it comes down to those really frequent touch points. So the beta we're running right now, again, I'm, every week, on calls with our users saying, " Did you use this? How did you use it? Was it seamless?" I'm looking for those cues about are the products we're building introducing friction? Where that friction gets introduced, that means that the AI or the UI, sorry, has to be adjusted to make it lower cognitive load, easy to understand, easier for people to adopt.
Jon Prial: That is such a recipe for success. So there is a couple of outstanding areas and projects that you've got some great stories about. So I'd like to start, have you talk about how Fiix and Georgians R& D team worked together. Then we'll drill into a couple of really cool solutions. But how did the process go, when you were working with Georgian's R& D team?
Frank Emery: Yeah. So actually, I think my first day on the job we met with Georgian's R& D team, which is a great way to start the job. Really, what it comes down to is Georgian's R& D team is there to give us that advice and help when we get stuck. So we have our machine learning team, we have two great PhDs who are great at this stuff. But at a certain point, you kind of hit this barrier where you need that expertise to help solve problems. I always think of it as you can be the best person at your job that you're going to be, and sometimes you need that fresh set of eyes coming in and saying, " Have you thought about this, or have you thought about that," to help you get through some really tough obstacles. That's really where we work with the Georgian team is when we hit a point where we need that external insight or that fresh set of eyes, they can come in and help us with that. Then also really hold us to task and make sure we're thinking about all the different problems at play, and where we might need to integrate a bit more support or more functionality into our product in order to make it that much better.
Jon Prial: Cool. So talk to me about where you are with the parts prediction app.
Frank Emery: Yeah. For sure. So that's the first product that we pushed into general availability back in July. So that's now live to all of the customers that we have that want it. It's actually scaling really nicely. So we finished our beta with a handful of customers that had worked with us along the way. I think we've tripled or quadrupled the number of users that are using it since then. So it's got fantastic market traction. People love it. It's actually really interesting, because a lot of the customers we talked to will look at this app, and actually see it as a catalyst to help them improve different aspects of their business.
Jon Prial: Can you explain a bit more exactly how the parts prediction, what it actually does?
Frank Emery: Yep. So what our parts predictor does is it learns the seasonal workload for how teams are operating, and what the inventory and parts consumption for that is. So we go into Fiix, and we can see all of the work that teams are doing, and all of the parts that you're using to do that work. So if you can think about it, when you're going in and you're fixing a truck, you're going to use some nuts, some bolts, you might use a new engine if it's really broken. We track all that stuff inside Fiix, and then what we can do is we can look at all of the work you're doing, the parts you're consuming for that work, and we can infer how much work you're going to be doing in the future. So using that, we can actually solve one of the big problems with supply chain management, inventory management right now is predicting spikes in demand. Because we can model seasonality and future demand for work inside a system, we can then actually predict where those spikes are going to be. Being able to predict where those spikes in part demand are going to be, we can give you way more accurate estimates than traditional forecasting methods. What we do is we take all of that demand forecasts, and we build it into a really easy to use support based on all of the stuff that you've entered into our system. So we get stuff like historical inventory usage, current inventory levels, minimum inventory levels. We take all of that data, the demand that we've predicted, and then we add it all together. We say, " Based on all of the data that you've given us, based on the data we've generated, here is what you should be buying in the next week or the next month in order to meet those spikes in demand that we're predicting."
Jon Prial: So prior to that, they were doing their best guess, they might end up having a need for a part that's not on hand. So I'm thinking back to your story about working with your customers. Here they are, people that are sitting with clipboards at best, and maybe pieces of paper in a file folder somewhere, probably not a spreadsheet. All of a sudden they're finding you telling them, " We recommend you purchase another 12 window springs." Is that how it works?
Frank Emery: That's not far off of it, actually. Usually, I think in one of our user's examples, they actually did have a clipboard. They were walking around the factory with a clipboard, and they were writing down their best guess as to what they had to buy. They didn't really have an insight into what was currently in their inventories. So when you're kind of leading with that approach, you're going off of luck at that point. So we're saying to them is, instead of just walking around with that clipboard and putting in random numbers, here is a really easy to use report, and you have two options. You can either buy what we're suggesting, or you can put that report next to where you're filling stuff into your clipboard, and use it to kind of inform your decisions. It was that second approach that is actually how we build a lot of trust in the product. So when we released it, we told people, " We get it. You have no reason to believe us yet. I understand. Here is the report, you go and you keep doing what you're doing, but put this beside your current work. Whenever you go and you purchase a part, look at what we're recommending. Take that into account, and then see what happens in the next week." We actually found that that was the easiest way for users to start using our reports. Then eventually they just gave up and they just started using our predictions outright, because we were so consistently beating what they had written on the clipboard.
Jon Prial: That's tremendous. So don't just trust me, I'll show you. Do what you want to do, just kind of keep your eyes peeled on the other side of this piece of paper. Were you able to take that data and actually, as you think about selling this to higher ups in the company, have an ROI, some types of returns you could tell the bosses that was coming out of all this data you gathered?
Frank Emery: Yep. So we went back, it takes a little while for the data to come out on hits. Obviously, it's long range processes. But for some of our beta customers, we were able to go back and actually look at the specific outcomes. For some of our customers, we were showing increases in forecast accuracy up to 80%, which is kind of crazy when you think about it. That translated into we're looking at 50 plus percent reduction in order sizes, actually. In some cases, 100% reduction of stock outs.
Jon Prial: That's amazing. So you mentioned stock outs. The other place working with the R& D team was about inventory. So you talk about stock outs. So how are the two related, and what was your focus on inventory management, and how did the data and the AI lead to improvements in inventory management?
Frank Emery: For sure. So the way this project actually started out was the team went into Fiix and looked at all of the inventory that we had. We actually noticed that some of our customers were having an epidemic of stock outs. So they completely ran out of parts, they weren't able to fix the machines that they were trying to fix. Then they actually had downtime. So they weren't even able to produce or ship parts at all. So that's kind of how this whole process started. So the way that we look at AI here is I think a lot of companies, they say, " Here is AI, what problems fit it?" Whereas what we did is we said, " What are the problems our customers are experiencing that you can't really solve with existing technology?" That stock out problem, and specifically inventory management, was the first one that came up. What we said was, we said, " Okay, well, if we can see that you're stocking out a lot, and we know you're stocking out a lot because you're not ordering the right amounts, how do we solve that problem?" It turns out that solving that problem means being able to have better accuracy when it comes to purchasing, better accuracy when it comes to figuring out the demand for parts going forward. That's really where we brought in that AI to say, " Well, with AI we can get you that accuracy that you need, so that you can manage your inventory better." Then there ended up being this really interesting virtual cycle where as soon as we started sending people these predictions, they said, " Well, if these are the predictions we're getting based on our current inventory management practices, imagine what we can do if we go ahead and invest further and make our inventory management even more robust than it is today, we can get even better accuracy, better purchasing, and save even more money, and avoid even more stock outs."
Jon Prial: Fantastic. So I'd like to close, we've talked quite a bit about the trust that you're building with your end users, these non technical end users as you prove yourself. The trust that you've got across companies, because you're keeping the data private, but you're learning from each company, and improving what you deliver to each of your companies. So how important is the message of trust at both the corporate level and at the product level?
Frank Emery: Oh, it's incredibly important. Whenever we talk to one of our really big customers about using AI, the first thing they always ask is, " What are you doing with my data? How can I be sure that it isn't going to help my competitors or put me at a disadvantage?" Some of the data we have, obviously, if it got leaked or jeopardized, a lot of our customers are in the same verticals or in the same spaces. That could be quite damaging. So if we didn't have a high trust environment, if we weren't able to prove that data was being used respectfully, I think we would have an extremely hard time getting anyone to buy into these betas. Because it is a big leap of faith from their part. They're saying, " Here is all of the data about how we manage our business from a maintenance perspective. We're giving it to you to hopefully make things better, but also understanding that it's going to be treated respectfully, and not leaking out all the company secrets in the process." So it really is hugely important to be able to have that trust with our customers, and know that we can approach them and comfortably make these asks, and have them say yes.
Jon Prial: Frank Emery, a fantastic story. A fantastic product. Great to see tech showing up in places where tech hasn't really been in the past. So it's been a pleasure talking to you, and I appreciate you taking the time with us. Thank you so much.
Frank Emery: Thank you for having me.
DESCRIPTION
You might think that getting your customer base to adopt your AI product is a sales and marketing challenge. But it starts much earlier in the product lifecycle, with empathy for your customers’ problems, creating solutions that fit into their workflow and building trust that you’re treating their data with care. Three pillars of AI product management. Frank Emery, our guest on this episode of the Georgian Impact Podcast, takes us through how Fiix is accomplishing this. They’re connecting teams, tools, data and processes to get critical insights, scale maintenance programs and boost asset performance.Today's Host
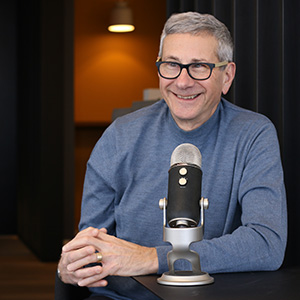
Jon Prial
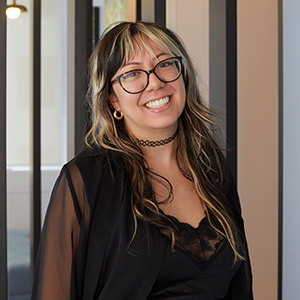
Jessica Galang
Today's Guests
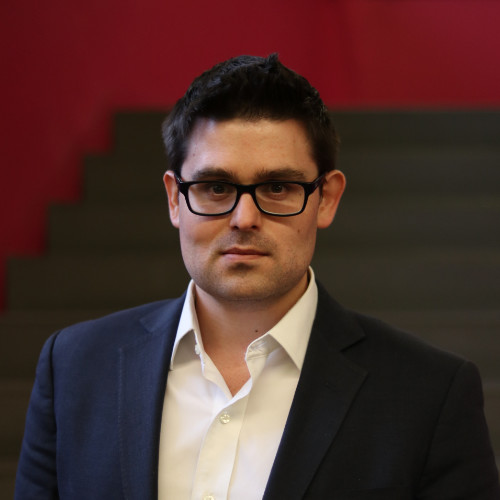