The Story of AI Transformation at BMO
- 0.5
- 1
- 1.25
- 1.5
- 1.75
- 2
John Fryer: What do you think of when you think of innovation, particularly around AI? I know some interesting software startup or maybe a software company that's hit unicorn status. But tell me, why don't you think about those big established companies that might not immediately come to mind? Well, if you don't think about these long- established companies, maybe a bank, you might miss an opportunity to understand innovation at scale, with complex technical and organizational issues too. Today, we're going to be talking with an amazing team of two, where many great folks of course, at the Bank of Montreal, BMO, one of Canada's largest banks. Not only are we going to hear about a great application, but we're going to be talking about scale. Remember BMO has a lot of customers and we're going to get into how a large enterprise can find that sweet spot between creating a small team of experts and building enthusiasm for merging technology across an entire company. Today, the story of BMO's CashTrack Insights, how artificial intelligence and organizational strategy go hand- in- hand. It's really quite a great story and I know you're going to enjoy it. I'm John Fryer and welcome to George's Impact Podcast. I see that a key to success in a large enterprise is clearly defined scope and skills with great teamwork. And this is where we'll be starting. The first voice you'll hear will be Peter Poon. Peter's the head of Canadian Digital Product Management, Capabilities and Innovation. And today you'll be hearing about digital innovation around transactional and day- to day experiences of customers. Also with us is an old friend, Yevgeniy Vahlis. Yevgeniy's the head of AI Capabilities. His mission is to bring AI across all aspects of BMO's business. So enterprises, almost by definition, you have to build complexity across an organization. There's the dreaded, but reality of a matrix. One part of an org has to care about product and they have their inherent challenges and development time to market. Another part of the business might be responsible for customers and revenue. Another might focus on operational excellence, but before you blink, there is a matrix they have to work together. So how did you get started? How did you really figure out how you're going to work this across the company?
Peter Poon: So I think it's an interesting lesson that we can take away. First of all, I think it's the confluence of several very interested parties who coalesced together to solve a key customer problem. Let me just give you some context on what the problem is. So first of all, day to day cashflow is a customer's top financial concern. Existing digital tools don't meet their customer needs. How many of us have used a spreadsheet to track our budgets or other tools? And we know that only 29% of them are actually happy with what they have. So since 2016, we did extensive surveys and found that consistently two thirds of our customers wanted help in managing their day- to- day cashflow. That is the number one concern and this challenge has been made even more significant during the pandemic. So furthermore, a third of our accounts face a cashflow shortfall during a year. So that translates to roughly about 2 million account shortfalls annually. So everybody faces it and it happens often. And historically, you'll see that many banks, we take advantage of a little bit of, I think by charging some NSF fees or other fees. I think it's a little bit of a win- lose approach, I think that regulators did look at. I think in the 2008 financial crisis. Frankly, there are banks that still pursue it. That being said, I think at BMO, we're really trying to do more good both in life and I think for our customers. And you can see that in our approach here, we recognize the opportunity for a win- win approach to cashflow, by helping the customers and then putting them on a better track to financial health. And fundamentally doing that, if we can translate all this data into an insight that could lead to a positive action from a customer's perspective, it could create a differentiated and a sustainable competitive advantage for the bank.
John Fryer: So the win- lose inaudible to win- win is you're acknowledging that although you make money on fees, you're really focusing on long- term customer loyalty and you believe this is one way to get there?
Peter Poon: Exactly. That's the way to grow the good with our customers. And frankly, we do believe that we need to provide value to our customers. And again, they'll reward us with their loyalty and with their business. And I think that's what we're here to do.
John Fryer: Tell me more about the survey. It's interesting that you were able to glean that this was a key problem for so many people.
Peter Poon: Right. And frankly, financial health is a key thing. There are a lot of emerging tools out there today. So we did three separate research periods with thousands of BMO customers and non- BMO customers in 2016, 2018, and from January to April 2020. Throughout, consistently during that time, we asked them a plethora of questions about what are their number one concern, whether it's retirement or day- to- day cashflow or investments, savings, borrowing. Regardless of, I think the period, it's still the cashflow, day to day, just keeping your head above water that is still being their number one concern. So based on that, we focused in on that element to tackle first and provide a real life solution to help them improve their financial health.
John Fryer: So Yevgeniy, you clearly have a ton of data, but as you start thinking about applying AI to this, tell me more about the approach you were taking. Were you determined that this was something that was a really neat application where AI would be the heart and soul of it?
Yevgeniy Vahlis: The challenge with AI in large enterprises is that there is a basic chicken and egg problem. AI is new. It's still new to the industry, even though we've been added for some time, we collectively. By and large AI is still a new thing. There are no pre- existing solutions that can meet every single needs that you can just deploy. And because there are no pre- existing solutions, there are no pre- existing patterns as to what you should be doing. The question is, what should you be doing? That's exactly the question you've asked. So there's no easy answer to that. There's a bootstrapping phase, which is, a meeting of the minds really, where you find the right partners understand the business needs, the client needs. And it's an iterative process, but it requires open- mindedness at every single step of the way, because you're creating something new and therefore you don't have necessarily budgets set aside to do that. It's not part of your roadmap because it's new. You didn't know it was possible. So you weren't planning for it. So data is almost like a... A lot of people will not like me saying that, but data is almost like the easy part here because it's there. There's tons of value that can be extracted from it. It's the connection between AI and the needs of the clients as Peter was describing. That spark needs to happen and you really need a great partnership for that to happen.
John Fryer: You say you evolve though, but it's amazing to me that your first customer set, which sounds like retail customers, is a lot. Were you nervous about tackling such a large use case in terms of how many users this would hit?
Yevgeniy Vahlis: No, not at all. Just kidding. We were all I think... And Peter can provide the product beyond this. We were all nervous, very nervous. And we did a lot of testing and I think the diligence of the team in testing the implementation, the technology, the messaging, everything that goes into it, paid off.
Peter Poon: Absolutely. I think it is very unique, pioneering new ground here, collectively, both from a product and an AI perspective. And Jonathan, there's two things that really make our solution unique. So, just to recap, it presents customers with an alert or a notification within the Bubble app. And it gives you a predicted amount and the timing of the cash flow shortfall and the recommendations on how to manage that. So this stands out on several fronts. So first, the notification and the calculation is sent up to seven days in advance of the predicted shortfall. We also tell you the size and the duration so that you know how to take appropriate action. That's very different than any other solution on the market right now, that may tell you maybe a few hours in advance. You may not even look at your phone during that time. You may not have time to actually deal with it. And not to mention, consider a solution that's right for you, or reach out for help to talk to somebody, perhaps an expert at the bank, to figure out what the best solution is, how to deal with it. So I think we're giving ample time for the customer to act on that recommendation, but not only that. Obviously, Yevgeniy can talk a little bit more about this, but the accuracy is very high in terms of how the model factors in the customer's holdings, but here's the key differentiator. We're assessing the prediction across multiple accounts, and that is a unique concept globally. We tested this very early. There was one gentleman who had well over six digits in his savings account, but close to zero in his checking account. So obviously he couldn't afford a inaudible that was incoming, but he had more than enough cash to cover it. So if we were to try to make a recommendation off of that scenario, it would be inaccurate, because the person had plenty of free cash flow that they were able to cover. So I think this is what's challenging most of the solutions on the market today. Is that they're not assessing across the entire holistic picture a customer has in terms of their financial holdings. And that's what we're able to bring and therefore yield a much more accurate and again, a correct path forward for our customers to say," Hey, we don't need to give you a lot of credit. It's just a simple transfer from your savings, checkings, and you're on your way and you're good to go. So I think that's what's extremely unique. And then finally, the third differentiating aspect of our solution is around the relatively strong customer engagement. As Yevgeniy mentioned, there's a lot of testing to go with it. There's a lot of very, very intricate calculations and modeling behind our solution and we have to ensure that it is explainable to our customers and at least they comprehend it and get an understanding of the smarts behind it. And how do you do that? We need to make sure that the verbiage and the context is provided. We need to show them the right amount, but without necessarily overwhelming them. So I think extensive customer research, thousands of hours have been put into it to co- create this solution with the customer so that we can develop and deploy a solution that makes sense for them.
John Fryer: I'm fascinated by a couple of elements of this. The one that's just amazing to me... I get the holistic view looking across all accounts, but the fact that you're able to look seven days out is really quite interesting. And then when I think about new apps, I often get hung up on the social medias that are doing dopamine rushes and they're like," You're not doing that. You're in the psychology of nudging." And there's been a lot of studies. If someone automatically enrolls in a 401k in a retirement plan, if a company automatically enrolls and there's a good deal, you have a much higher take- up rate than not. If you put water bottles near the register and the soda cans in a machine somewhere far away, kids are going to drink water. Now there's this subtlety of nudging. So how did you think about people's decision- making? I got the facts and I got the data led to that, but I'm really quite fascinated on how you're able to present this and tell me... I assume you've got happy customers with us. So tell me a little more about that.
Peter Poon: Sure. And again, to your point, the nudge theory, it matters and it works. There's a lot of research that points to that way and I think the key is to how to apply it. You don't want to come off like a nanny, like a wet blanket because we bankers... And I think this is a really key lesson for a lot of folks in VinTech too. We wake up dreaming and breathing VinTech, but 90% of the population outside of us, they don't. So when you're here to remind somebody that they've got a cashflow shortfall, it's not necessarily a fun message. So you need to be very respectful about how you deliver that message and again, sensitive to their needs and understanding what the context of that message will be. And then, not to portray a doom and gloom message, but there's help available and there's a path forward. And I think that's where through a lot of customer testing, we hit on the right tone to say," Hey, look, this is what's coming up, it's not the end of the world. This is what's going to happen, and this is where we can help. And this is what you can do to get there." So I think just delivering an even keeled tone and also providing a solution that weights through all the data for them, rather than having them to do it themselves. I think that's going to be key.
John Fryer: And it sounds very empowering. And you mentioned the data and Yevgeniy, you talked about the data being the easy part, but one hard part about the data, talking about how you manage and you were disclosing issues of privacy, are you doing anything with looking across accounts and aggregating data? Tell me more, a little bit about the data, even though it's easy to you, but not to me.
Yevgeniy Vahlis: It's not that it's easy. So, just to clarify that point. It's extremely hard, but it's hard in a different way. The inception part is hard in a way that's completely unpredictable, whereas the data is hard in more predictable ways. But just to get to your point on privacy. So the way we've set the strategy for AI work at the enterprise level is we don't work with personally identifiable information at all. We don't touch it ever. There are extreme situations where it's necessary to look at inappropriate amount of personal information with all the necessary approvals to make sure everything is working correctly, but the work and the models and the solutions just don't touch personal information. And that's one of, I would say, the beautiful things about using AI. It doesn't need that information. It's all about the aggregate, the statistics and the patterns that emerge from the data. The data itself can tie back to Sudan and most entities, which we know nothing about beyond what we're seeing in the data itself. And then the actual end systems that expose the information to the customers, those systems are the only ones that know who the information really belongs to. So I think it's all designed and architected in a very healthy and privacy preserving way. Now beyond the technology, there's also extensive governance around access to data, the use of data for various purposes. There's a trustworthy AI council, which we're both members of. It's a collection of leaders from across the company that sets the tone and the strategy for the organization around how do we make sure that AI is used in an ethical, privacy preserving way? It's one of those things that's an enabler. If you set privacy up from the very beginning, you clear the path for yourself to do really great work. If you don't think about privacy at the very beginning, then it comes back later on and becomes a major hurdle for any initiative.
John Fryer: So I'd like to wrap on product. Peter, I've seen some of the data, but could you share with me some of the activity results you've got deploying this product since you've launched it?
Peter Poon: From a customer perspective, the response has been overwhelmingly positive. They're rating Insights and the overall cashflow prediction, an average of probably four and a half to 4. 6 stars out of five. If it was a separate app alone, that would find us right near the top, if not at the top of the app store. So it's fantastic customer feedback. But also from an engagement perspective, when we push out and when we share the recommendations for our customers, they've engaged with these recommendations well over the industry average of close to 1. 5 times higher than the industry average. And furthermore, I can loop in back to the privacy aspect and the data aspect. Our position is that the data is always the customers and what they choose to do with it, it's always in their hands. What we're trying to do is simply show them the data that they have, but also provide them a little bit better insight so they can do more with it. And that's really the approach. But we always give them the ability to opt out. And so with that, I think less than 1% of our customers have opted out of the overall Insights program. So again, it shows, I think really strong engagement from a customer perspective. Furthermore, I think longer- term too, as we add more robust recommendation capabilities, we're tracking well towards upwards of$ 7 million revenue opportunity over the next five years generated by a combination of products and which customers will choose to avoid cash flow shortfalls. And you can imagine that the plethora of loan products for credit products and overall, frankly, overdraft protection and other products that we can share with our customers to help them out of their immediate cashflow shortfall. So that we're tracking well there and lots of opportunity to come.
John Fryer: That's tremendous. So let's talk about organization. The challenge a large enterprise has, that probably any organization has, is you always want to have your your center of excellence. I'll use that term. Your AI team in this particular case. However, when you have that team in place, it's quite often for other parts of the organization to abdicate and say," I'll leave it to them." And if that's the case, you don't scale, you can't do enough, you need buy- in, you just can't do it alone. And yet you can't disperse your resources everywhere because there aren't enough of you to disperse. So how do you balance between creating that competency center and getting this bought in across the company?
Yevgeniy Vahlis: Maybe as a bit of background. BMO has quite a few, I would say, Centers of Excellence across the company on various topics, but, analytics and AI in particular. The AI capabilities team is not a Center of Excellence. It's a product team. And so the way we operate is actually very familiar to you and to George and partners. We built to scale. So we built essentially SAS Solutions within the company. These would be services, products that deploy within other systems, products that deploy within systems that the AI team operates. And we scale in a similar way to how a SAS startup with scale. We built once and we make it available to everyone in the company. And so that's the difference between a COE and a product team. A COE operates as a consultancy and there the scaling is linear. So for every hour you need to put in it's an hour that you need to put in. Whereas a product team tries to scale by building a generalizable solution and then making it available to everyone. And so a lot of our consumers are actually the COEs, as you mentioned, and they're the end users for the capabilities that we built.
John Fryer: And you're vying for attention then. You're not the only product team in the company. How do you get their attention? How do you get them to again, to provide valued insights to you and as Peter mentioned surveys, but how do you establish your working relationships with these teams?
Yevgeniy Vahlis: There's a number of aspects to it. There's initiatives like the CashTrack work, which really bring it to the top of mind for a lot of people and they start thinking about what else can you do with AI? That's one thing. You just have a few successes that can demonstrate the capability. The other aspect that's been very helpful is really the fact that we would focus on capabilities. I use that word a lot, but it's really what we do. It's things that were not possible before and yet are beneficial to one or more teams across the company. And so the way you partner up and the way you get attention is, you actually bring good value to those teams. You enable them to do things that they couldn't do without you, and you don't engage in work that can be done in simpler or different ways. And so we're a small team, but we rent there with an enterprise- wide mandate. And so we're really focused on that, and the most impactful and reusable capabilities, I would say.
John Fryer: Do you get people knocking on your door? Is there a little fear of missing out here once you've got a couple of successes under your belt?
Yevgeniy Vahlis: Yes. We've been fortunate in that sense and a lot of it is thanks to Peter and his team because they were the first ones to bet on this new AI Team that was established a couple of years ago. And through some of these initial successes, I think we built some good momentum for AI at BMO, overall.
Peter Poon: I want to just take a moment to build on Yevgeniy's point around the organizational hurdles. I think across the board, it's very interesting, obviously not only at BMO, but at any large organization, there's a lot of number of teams that you'll have to first make that connection and partnership. I think that's the first piece. But also to find a way to incubate and develop the concept. Yevgeniy's Point, it was a new concept. It wasn't fully funded yet. There was a lot of groundwork that we needed to do and experiment, frankly. We're very fortunate. We have a very well- established digital innovation program called BMO Innovate, and so there's a well- established framework and pipeline. One of the themes that we chose to explore and invest in was around, I would call it the maturation of data into Insights. Banks are swimming in data as are most of our customers today, but there's a broader struggle in terms of what does this mean for me as a customer? And so that was one of the themes that Innovate chose to explore, and this fit that thesis very, very well. So when we partnered with Yevgeniy on that, I think this was one of the first items that we wanted to test and through various light iterations of testing, we developed the model, we tested with customers, we upped the accuracy, played with the refinement of the concept, and that's where we were able to gain enough confidence in it over time to invest more fully in it and in a scaled up solution. And that's where the core team then stepped in with embedded numbers of Yevgeniy's team and embarked on a full agile deployment process in which we launched it to market across millions of our customers. So I think that's the broader story. You've got to have a good framework to experiment, and a safe space to experiment in a time- boxed way, so that you can understand what the key takeaways are. Refine the solution before you scale it to the masses.
John Fryer: I'm hearing funding, I'm hearing testing and scaling. This is great that you're built it all together. So, if I understand this correctly, CashTrack Insights is part of a broader branded offering, is it BMO Insights? Or you've been asking... BMO, my apologies, I'm American. Let's talk about the future. What use cases do you see coming next?
Peter Poon: Oh, wow. The sky's the limit. Obviously, Yevgeniy's got his magic workshop there, ready to roll, but for us, I think it really anchors around the theme financial health. So, I think the first thing is helping customers just get their head above water from a cashflow insight perspective. So helping them spend, helping them just budget and save, that's the first piece. And then the next part is, how do you help them accumulate? So if you think about spending, not savings, how do you help them accumulate the right amount so that they can build a little bit of a cushion at least? Especially during these perilous times with uncertain economic climates, it's very important to help people build a nest egg. And I think there was another older survey of 40 to 50% of folks couldn't afford an unforeseen expense of four to$ 500. And that was US figures, but if you translate into Canadian, that means if you popped a tire. You popped one of your tires, you would have problems simply going in to fix it and replacing your tire. So it's a very, very tough situation to be in. So we want to be there to help, and I think that's probably going to be the next area of focus. Just, how do we help our customers start saving better? And that goes right back to your point about nudging. We don't want be the wet blanket, but we want to be able to show our customers how easy it is just to do a little bit a day and to help at least pad and establish a little bit of cushion so that when the unforeseen thing happens, that they're ready for it. I think that's going to be the next step and then more broadly, we will probably expand into more longer term financial health themes, like investing and retirement, et cetera. But I think the first next step for us is going to be saving. So automated savings insights, automated savings transactional transfers that would happen. So I think that's the theme that we'll be embarking and exploring a little bit more. Yevgeniy, anything else on your end?
Yevgeniy Vahlis: One other thing I'll mention, and that's something that we've done differently at BMO relative to two other FI's. AI has generally picked up momentum in Canada in the financial space. And I think one of the things we've done differently is really the constant strive to get AI in touch with the product. We didn't start as a fundamental research team, even though we have those capabilities. The backgrounds of the team members, they're all PhDs and masters in Computer Science inaudible. They've all published at the top AI conferences, but we've from the very beginning, tied them to the product through this collaboration with Peter, but now with other groups across the company as well. And if you look at just the speed of delivery. If you compare us to other FI's, we've delivered collectively as a company, in terms of AI initiatives, quite a bit more, just over the course of one or two years and previous to that as well. But I'm thinking about them and the way I see this evolving is we'd like to continue with this highly applied, highly productized and productionized approach to AI, because that's the part that's really hard with AI today. It's not that the science is easy. It's just, we have people who got this, they're very smart. They're experts. They know how to do it. They'll figure out the science and we hired them to do that. But the productizing of AI is hard and it requires collaboration between teams like Peter's and teams like the AI team. And so establishing that model, scaling it up across the organization, that's I think what the immediate term looks like.
John Fryer: That's a great story. I'll close on Peter's example of the popping a tire. You guys started where the rubber hits the road, tongue planted for only in cheek, but it's a great story. It's a great balance of product and customers and needs. It is terrific. Thanks so much for spending the time with you. Peter and Yevgeniy, it's been a pleasure. Thank you.
Peter Poon: Thanks for having us, John.
Yevgeniy Vahlis: Thanks, John. [ music ]
DESCRIPTION
Peter Poon and Yevgeniy Vahlis are our guests on this episode of the Georgian Impact Podcast. Peter is the head of Canadian Digital Product Management Capabilities and Innovation at BMO. Yevgeniy is the founder and head of BMO AI Labs and they will show you how a large enterprise can find that sweet spot between creating a small team of experts and building enthusiasm for emerging technology across an entire company.
Today's Host
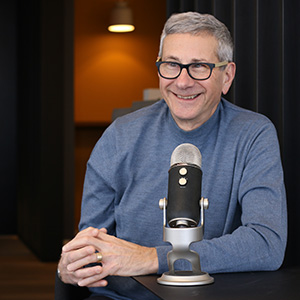
Jon Prial
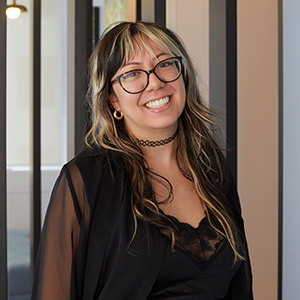
Jessica Galang
Today's Guests
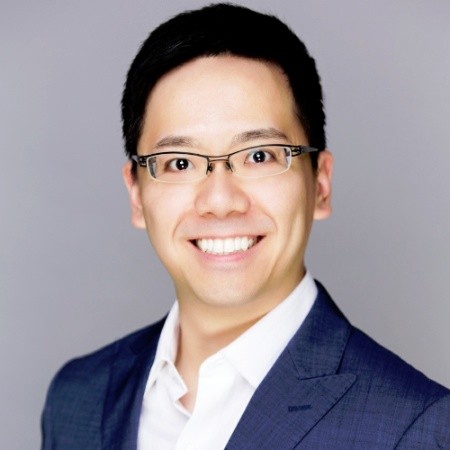
Peter Poon
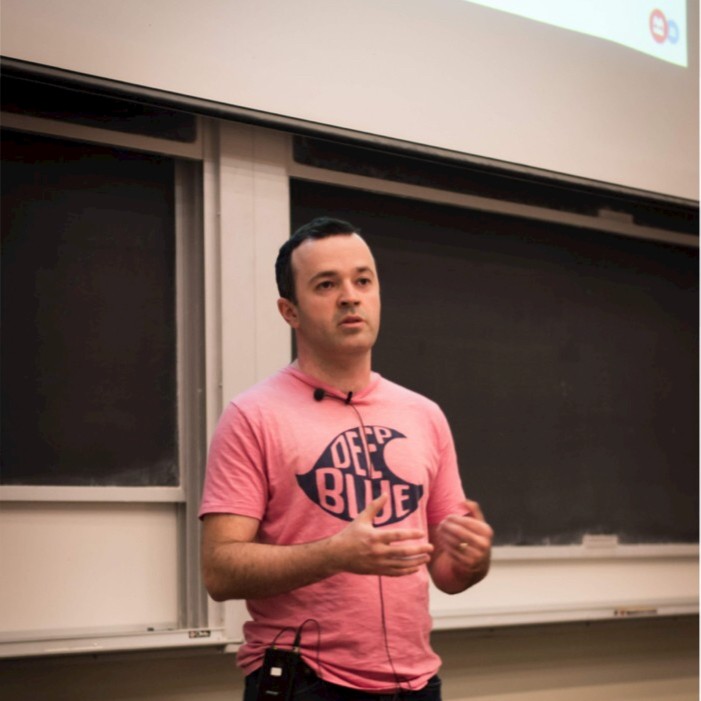