Getting Conversational AI Right at Microsoft
- 0.5
- 1
- 1.25
- 1.5
- 1.75
- 2
Jon Prial: Welcome to Georgian's first podcast of 2021. Now, we've always recorded our podcast remotely and we've taken advantage of great tools. We absolutely consider ourselves so fortunate to be able to connect with such great guests this past year, but we are happy to be entering a new year with renewed optimism. What's coming? More of the same, but we are looking to organize small series of podcasts around single themes. So let us know at podcast@georgian.io what you're interested in. We love getting feedback. We here at Georgian have spoken and written a lot about having diverse teams, particularly around AI. The evolution from the development of algorithms to feeding huge amounts of data into models has put us in a new world. Now, there's great opportunities for companies like yours, but these opportunities come with challenges that must be proactively addressed. See, having a problem down the line with biased outcomes or unhappy customers makes recovery a challenge. So we recommend getting started on the right foot. Today we'll be talking with Deborah Harrison from Microsoft. Now listen carefully to her title. She's a senior content experience manager in an organization called content intelligence. Content intelligence sounds to me this is the foundation of what makes user experience work. We all know of UI and now UX, and we know that there are a lot of users out there in the Microsoft world and your world, too. So we have to make sure your thoughts on diversity get into that foundation, providing your customers the right experience, and that is down to the content as much as the technology ensures you become that trusted resource to your customers. You might've guessed that Deborah was part of Microsoft's Cortana team, but there's more. It's time to understand how your content is your brand, your personality, and it's more than linguistics, too. There is a lot to unpack here, and I'm looking forward to a great chat with Deborah. I'm Jon Prial, and welcome to Georgian's Impact podcast. Deborah, big company, fascinating job. Tell me more.
Deborah Harrison: Oh, you bet. My team is called the content intelligence team. We're a team of writers working in a design heavy, thoughtful space, and we focus on we're sort of first among equals. We sit in the windows and office and that world, but we work with teams all across Microsoft. And as you mentioned, my background is in Cortana, and so my team has collected a lot of expertise in conversational design and conversational experiences. And so that's been our bread and butter for a few years, but as we've worked on more and more projects across Microsoft and with partners, we're seeing this common theme that shows up more and more prominently, which is that conversational experiences and other experiences that have whether or not expressively dialogue driven design continue to have this need and this greater presence of building the machine learning and the AI that underpins those experiences and that they need to have a very human driven, ethical, thoughtful approach at the same kind of approach that we bring to our conversational design work. And so my team has developed this specialization in this content intelligence universe, and we work on a variety of projects that draw from the work we've done that helps us understand how to articulate and, as you say, operationalize, to some extent, the factors that contribute to an inclusive or ethical, which I realize is a very big word, but I use advisedly in this space, experience that a person can have with the device or feature that they're building. So we are writers. Our area of expertise really comes from using words.
Jon Prial: Human driven approach to machine learning and AI. This is just gold. Deborah, you were one of the original architects of Cortana's personality principles and served as the first author for all of Cortana's dialogues. Now, we've heard about the woman that was hired to be Siri's voice, but I want to hear about the person behind Cortana's personality.
Deborah Harrison: When I started on Cortana, I was the only writer. It was just me and it wasn't a team at the time. It was one feature on Windows phone and much like all the other features that any of us writers had worked on before, where we were just sort of parceled out between the things we were expected to do. So I had accounts and email before this, and it would be the out of box experience. And so with Cortana, it became apparent almost immediately that the models we'd been developing to create this much more humanistic, casual, conversational language that we were able to view Windows phone with, even with the amount of casual and conversational, we were encouraged and, to some extent, developing the ability to be, it wasn't going to work for Cortana because Cortana has this identity and agency and this sense of persona. And so it needed to have a specified voice. And that hadn't been thought through yet because the writer hadn't yet joined the project. And so I did some thinking on that. I collaborated with some other folks who were in the design world who were thinking about it from an avatar perspective and from the voice hirings. Did the voice talent perspective and those areas, and we put together some guidance that helped shepherd that. And then over time it became clear that one writer couldn't even begin to do the amount of work that needed to be done. And so gradually a team formed.
Jon Prial: It's so clear you couldn't do this alone. How did things evolve?
Deborah Harrison: My manager, Jonathan, who came onto Cortana to lead the team and became my manager then, and we've been working together ever since. It's been an extraordinary partnership. And he worked with, at that point, the two of us writers who were there to begin thinking about what is it going to take to do this work? And to some extent, we were working backwards. In some extent we were thinking forwards, but Jonathan was really resolute about the need for people who had experienced embodying a persona and a personality and the thought process of the dialogue, which meant functionally that the team became built with people who had these liberal arts backgrounds and these humanities backgrounds. And so the makeup of the Cortana team became people who were musicians, who were poets, screenwriters, playwrights, novelists, people who had this experience of trying to put themselves within the embodiment of another person and be empathetic.
Jon Prial: Again, the humanity comes across. I really, really like this. When you described your role at the beginning of the podcast, you mentioned having a greater presence in the building of ML and AI experiences. Now, I know semantics have always mattered, but I'm realizing that they matter even more as you and I talk. I think it's great that you have a breadth of touch points across the company. So how has your career and in parallel awareness of all of this evolved?
Deborah Harrison: You know, I'm always a little bit wary of the things that are different now than they were before. A lot of things are on a continuum and has as much to do with your perspective. But I do think that in my job 10 years ago, before the prevalence of ML and AI within any given feature, certainly anecdotally, I wouldn't have been having these conversations at this level, nor would my peers or my team be expected to do that. And I think of that as one of the great fortunes of the career that we find ourselves in, that we get to reckon with these incredibly weighty, meaty questions of," What does it mean? What are we? Why don't we tell all our machines to be what we are?" And yeah.
Jon Prial: I think of all the goofy things that people just try to do with these virtual personal assistants. Asking one to marry you, asking ridiculous questions, or even tossing an insult at this device or app. How should it react to something negative, maybe being called fat?
Deborah Harrison: Fat is a great example. I'm just going to cue on it, because you just said it. So I can't speak to whether it's clever enough to meet the bar here of this conversation, but that was a really good one, because people say," You're fat," meaning it as an insult. We understand it. We don't have to interrogate that consciously. And yet if we responded in a way that keyed off of the insulting factor of it, in some ways it validates the idea that being called fat or calling somebody fat deserves to be an insult, which we reject. We don't agree. And so we wanted to find some sort of response that intelligently acknowledged the likely intent of what the person said, but without tacitly endorsing the concept that fat is a negative or that it's an insult. And so I'm trying to remember. I think we actually shipped two or three different responses to that, but one was, I think it was," I'm comfortable with my curve." And Cortana is a round symbol. But we wanted to say something that wasn't like," How dare you," or," No I'm not," or something, a denial. Or," That's rude," or something, because it felt like it would offer power back to the idea. So that took us weeks.
Jon Prial: Well, I really like this because, how to get it right is much more complex than I thought. So can you and I go back in time? Not too far. I mean, if we want to go really far back, some of us might remember Microsoft Bob. That was added to the early Windows platforms to provide a user- friendly experience, and that didn't work. If anybody wants a chuckle, go search it out. But let's you and I talk about another conversational helper, if I may. Microsoft Clippy. Did you look at that history?
Deborah Harrison: Yes, of course we did. Of course we did. First of all, no, we decided that Cortana was going to have a lot of affection for Clippy in a similar kind of way that people... It's completely reasonable for people to have their very legitimate frustrations with how the experience was using Clippy. I was frustrated using Clippy, too, but most people come to Clippy now as a retroactive thing. They didn't actually use it, and it's more of just a punching bag. And so we decided really early on that Cortana is not going to insult anything. Cortana's not going to be mean. And so we developed a string of responses, which honestly, nobody asks that much about Clippy, but we inaudible. We developed this backstory that Clippy's retired and living in Boca Raton and perfectly happy. And that was inaudible Cortana. But in terms of the work, this came up constantly. What does it mean to be Clippy so that we can't be Clippy, so that we aren't Clippy? And one of the things that I come back to constantly, and I don't work on Cortana anymore. It's handled by a lovely team of incredible people who are still working on it. So they might speak slightly differently to what's happening now, but Cortana is kind, and I'm not saying they don't still embody that, but Cortana also needs to be intelligent.
Deborah Harrison: I have socks that say," It looks like you're trying to get dressed. Do you want some help with that?"
Jon Prial: Context really matters. It sounds like the techies were just being too cute. And I'm probably going out on a limb maybe, but I'm going to guess it was the techies, just the techies in a room. No one with the skills that you and your team have were even near the building. So my view of Clippy is that it was there to answer questions, but its view of," Oh, I see you're trying X," wasn't all that powerful.
Deborah Harrison: And so one of the things that Clippy did poorly or ineffectively was to misanticipate what you needed. And I see this in the development of machine learning and AI to this day all the time. That we are very excited collectively about the idea of anticipating a need you might have and then trying to meet it for you before you even knew it. There are places where that's very effective and it happens without our noticing it all the time in the places where that development has gone to a healthy place. But especially in the conversational space, where we don't want to be wrong, the temptation is to guess and then ask. And what I always try to recommend, unless the confidence has already been built and the level of trust has been well- established, which can be done. You can do that over time. There are models you can build that anticipate the level of trust that's been established and then allow your agent to speak accordingly. But in the early stages of that trust, what I recommend is asking what you want, rather than trying to guess.
Jon Prial: That's a great point on building and/ or breaking trust. Recognizing context requires more than just where you are with the current usage of the app. Questions, issues can come from most anywhere. So we should think about balancing understanding and managing people's bad behavior or limited thoughts that we mentioned earlier with giving customers what they want, and then we're on a good track.
Deborah Harrison: That's exactly right. And I think those minor places carry with them, to go back to what you were saying before about prejudice, carry with them a set of assumptions about what constitutes minor. And I think, I mean, this speaks to hiring as well, and it speaks to the makeup of your team. My team, there's diversity represented on my team. That exists, but all of us have chosen to live in the Pacific Northwest and work at a multinational company, and all of us are bringing those sets of biases into our day to day. The idea that a bot can be or a conversational agent could be, say, race neutral or gender neutral is, I think, a tricky concept at best. And I think when we think about what constitutes a minor misstep, I think our biases play into what constitutes minor as well. So if I'm anticipating... This is an example that comes up reasonably often, I hear, in anticipatory development. The song example is a great one. So it's not just," Do I want to pick a song for you?" It's," I can." Because we can track, because in the industry it is possible to pay attention to things like mood or affect, the temptation is then to take that and label the affect and then assume that you can respond appropriately to that affect with something that somebody wants in return. And so the practical upshot of that would be something like," I can tell by the variety of signals that you're giving off that you're angry. Let me recommend a playlist for you." Well, what constitutes what do I want? Let's say you're right. Let's say I'm angry. Let's say I'm super enraged about something. What I want to do about that rage is a very personal choice. Am I righteously enraged? Well, I don't want to be calmed down.
Jon Prial: I like that example of music selection. I might not like punk or play soothing white noise, but I do have a range of music that I like to listen to, and it is based on my mood. So I'm really glad to hear this. So let's broaden our discussion a bit. How do you view emotion? Is it a bias that you actually need to consider as well?
Deborah Harrison: And so the assumption-
Jon Prial: crosstalk.
Deborah Harrison: Yeah. And that might be something that I don't even admit to myself, because I have some biases that I've brought into that conversation. And so in that circumstance, trying to anticipate carries with it the potential almost for it could feel offensive. I recognize that's a giant word to use in this case, but I feel like the expectation that you bring away from that is not like," How dare you," as much as like," You don't get me at all." You know? And so to do the kind of work that we do, which to be clear, I'm not actually in the predict your music business as much as I am in the think about the words that we put in the mouths of the agents that we've devised business. When we think about that language, we are thinking about it from a very particular point of view, a very well socialized point of view, one that feels invisible without arbitration. And so part of my job when I'm hiring or in my case, it's been quite a while since we've had any open head count on our team, so therefore we have to think about how do we bring people into these conversations? How do we create conversations that challenge our own assumptions and biases on a daily basis and with safety and clarity, but also with challenge? So that's part of the role. And then the other part is how does it not become an insular conversation that only resides within the echo chambers of our own group? Because we construct our own limitations and biases over time.
Jon Prial: So staying with this topic and talking more about bias, so good AI or great AI has a lot to do with training and continuous learning. And you did have some prior markets of glitches with a conversational bot that I'm sorry to bring up bad news, but I'd like to talk about it. Tay, and even her younger content- free apolitical sibling, Zoe. How did you put up guardrails?
Deborah Harrison: Yeah. So with Cortana and then with some of the conversational agents that we've worked on in the wake of learning about what we learned from Cortana, the personality chat work that I took on after that, we actually, upon launch, we would have people... We hired a vendor team with the personality chat launch to do 24/ 7 monitoring to see partly it was in anticipation of the likelihood of being brigaded by 4chan and other hostile actors. So nobody tried to brigade our personality chat. Now I've said it out loud. Oh, no. But so far no one's tried to do that, so that's good news. But what did happen because we were doing 24/ 7 monitoring is we were able to pay attention to... And to be clear, this is entirely anonymous. We have no idea who says what, right? This is not tracking who says anything, but we do have insight into what is asked. And we found tons of examples of things that we had either just it hadn't occurred to us that people would ask it, or we saw it in a different light because of how somebody responded. You see the chain that emerges after it. Or as often as not, something would misfire and the misfire would be catastrophically offensive, because it is really misunderstood. And so we had sounds kind of more strategic than it really was. We just did. There are just a few of us whose job it was to pay attention to this stuff. But for things that were really bad, we would handle it right away. We would go in and fix it.
Jon Prial: So to wrap this up, let's talk about a slightly different aspect of context. It's about helping your users with their grammar, their writing style, suggestions. And I still don't know that I like it yet. Now, how important will that be on the human side or even on the computer generated side?
Deborah Harrison: And so that's one of the things I always recommend if people are doing anything in the conversational space, but really this is true. So a lot of the work that my team is doing right now, we're doing a variety of projects with an editor, which is a feature in Word and on a browser where you can ask Microsoft to offer you suggestions about your writing. And so some of it might be in the realm of spelling or grammar, those squiggles that we've been familiar with for decades now. And then we continue to evolve the places where we might be in a position to offer some insights or help. Well, the unintended consequences that can occur by juxtaposition or misreading there can be pretty severe and pretty disruptive for people, especially for something as personal as their own writing. Right? And so that's the thing we're paying attention to is how do we respond? Not just quickly, because I think that's a thing we can definitely do. We have methodologies for dealing with problems. But how do we also let people know that we heard you?
Jon Prial: Right. Isn't this the best open- ended conclusion to a challenging space? We hear, but we need to listen. We need to understand and empathize. Deborah, you've been doing this for over a decade. We've evolved quite far, and it's just going to get better and better with people like you on the case. Thanks so much. And for Georgian's Impact podcast, I'm Jon Prial.
DESCRIPTION
When AI needs a personality, you need a team of creatives to work hand-in-hand with the technical folks.
Deborah Harrison, our guest on this episode of the Georgian Impact Podcast, is one of those creatives. She talks about how she and her team developed Cortana, Microsoft's conversational assistant.
You’ll Hear About:
● Deborah’s role as Senior Content Experience Manager and what her Content Intelligence Team works on at Microsoft.
● Deborah's work at the author of Cortana's dialogue.
● The evolution of Cortana’s team into a diverse group.
● How previous Microsoft conversational “helpers” influenced Cortana.
● The importance of asking, instead of anticipating in early stages in order to build trust.
● The need to create conversations that challenge assumptions and biases, to avoid insular conversations.
Today's Host
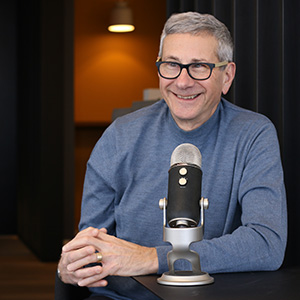
Jon Prial
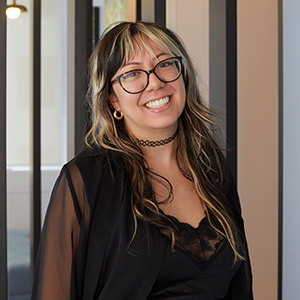
Jessica Galang
Today's Guests
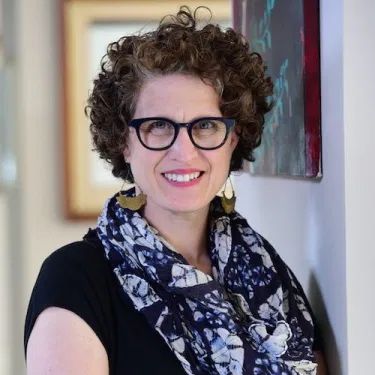