AI in Business with Emerj’s Daniel Faggella
- 0.5
- 1
- 1.25
- 1.5
- 1.75
- 2
Jon Prial: I could probably rattle off a lot of phrases that bug me mainly because they're overused, they gloss over the important bits. And here's one that might be waning a bit, but it's a good launching point for today's podcast. It says just take X and add AI. I mean, who doesn't love a little magic? But I think the reason the phrase is waning is because we're collectively recognizing and settling into the notion that the data that we have is truly changing much of programming, and that machine learning is a critical piece in successful software development. And with ML becoming pervasive not much more can be done. So let's just call it AI, shall we? So when? How? Where? We know deep learning isn't for all, and some of the more basic ML techniques are sufficient. But all software development, all product service creation comes down to cost to produce or deliver against the revenue in the market. So today we'll be talking with Daniel Faggella, CEO and Head of Research of Emerj Artificial Intelligent Research. Emerj is a research and advisory company working with business leaders to help them develop winning AI strategies. What makes this more fun for me is I get to turn the tables on Daniel. You see, Daniel's the host of Emerj's AI in Business podcast. Practical, actionable interviews to help AI leaders thrive in disruption. We'll put a link in the show notes. I know you're going to enjoy it. So Daniel, welcome. Emerj recently published a report on finance industry and AI. What are some key takeaways? Glad to have you here.
Daniel Faggella: Yeah. Hey, happy to be here. So the report itself sort of broken out across the two major sectors where we work. So most of our clients are either going to be in banking or in insurance. So we do work in wealth management as well, and that was rolled up under banking. But it's really those two sectors with the preponderance of our private sector clients. We do a good deal in security as well. But the main takeaways for us were really around where money seems to be being invested and why. We ended up getting some great access to heads of AI at places like HSBC or US Bank, or C- level folks at Citibank, as well as some 120 vendor companies, to get a sense of where the heck are people spending in these big sectors? Well, we know there's money flying around. We know there's press releases flying around, but as we found out the difference between what the press release is saying and where the money is going is exceedingly stark in finance.
Jon Prial: Interesting. I hadn't thought about it. I love obviously, the constant following the money is perfect. So what's happening at the high end, the bank spends? And what do you think is happening on the suppliers of the tech?
Daniel Faggella: Yeah, so if we look at big mega trends that are really important for people to understand, one of them is around the relative unfundedness of customer- facing anything. So when I mean that, I mean customer service applications, marketing applications, sales applications. While something like 35% of the outward- facing banking press releases about AI have something to do with, let's say, chatbots or customer facing something, there's a minuscule, less than 20% of the funds that we estimate are being invested by these substantial companies. Most of this was global 50 firms. We had a big focus on the top seven US banks by revenue. Something like less than 20% is actually being invested in terms of dollars there. So I think a lot of people think the customer experience is being overhauled. We actually realized a lot of reasons why,( a) banks are being misleading about that and why they're saying they're putting a lot of attention there. And( b) why that area is so hard for banks to actually improve? So we can talk about where the money actually is going but one thing that's often very shocking when we talked to folks in the boardroom at financial services firms, is the fact that what they thought was all hot to trot is in fact, really not all that much progress being made, relatively speaking.
Jon Prial: Wow! So, I'm fascinated. I do think a chatbot is the bright, shiny object that they're chasing after. And I haven't found one I like yet. I think there'll be a big deal at some point once they figure it out. But I love your thoughts on what's not happening on the back end, and yet we hear issues of bias and processes that are still cumbersome. So what does the CEO think of when he or she is making the decision as to where to invest?
Daniel Faggella: Well, this is the challenge. So you're taking me in an entirely different Pandora's box, but I'll open whatever box you want to open here so long as it's in my purview. If it's not in my purview I'll tell you. But that question is a rather grand question, and that's the crux of the business here. So when a CEO is thinking about AI, unfortunately, John, at least as of today, they're thinking of AI like IT. Often they're thinking of it as something that can automate things. So AI is IT, when in fact, the real sea change that needs to occur is three- fold. We have a great article called Executive AI Fluency, which is probably Googleable. If people search up emerj.com/ executiveaifluency.
Jon Prial: And we'll put a link in the notes for sure.
Daniel Faggella: The general idea here is that we need leadership that understands conceptually what AI is, how it's trained and what it can do. We need leadership that understands some representative set of use cases. They have a bs- o- meter, if you will, and a reasonable understanding of what it can do. And thirdly, they need some kind of a realistic understanding of what the roadmap to deployment looks like and the real challenges. Now, the fact of the matter is in the C- suite we essentially have almost none of all three. And so really it's not what does an exec need to think about? It's really about bringing folks a little bit up to snuff on context so that when we show them use cases, when they see the landscape, they have a more realistic understanding of where they might want to invest. So one of the big changes there is AI not being IT. Now I could double down on that if you'd like, because I think that's an important point.
Jon Prial: Please do. I think that's fascinating. I love the concept. I think that's great.
Daniel Faggella: Cool. And the exact phraseology of that, I will lend credit to a fellow whose been an advisor to me for a long time by the name of Charles Martin. He's a PhD in the physics space, but he's been doing AI consulting with big companies like Black Rock and eBay and whatnot for the last 10 years. He's in the Bay area. I lived in San Francisco for about three years until a couple years back when I moved to the East coast. This is where our customers are ultimately. But AI is not IT is an important concept. Here's the deal. With IT we really need to spec out what we want it to do. And then we need to figure out how to integrate it. And then we need to make sure people are trained on it. And then we can make it work. So,'Hey, when we push this button, we needed to do this thing, Hey, when this message comes in, we want this thing to happen to it."" Okay we'll build it out in the software." AI, however, is a lot more like science. With AI we don't know that when we push X, Y is going to happen. We have hypotheses. We suspect we could inform this decision this way. How are we going to find that out? Well, we're going to need to fiddle with the data. We're going to need to talk to subject matter experts and understand the features of that data. We need to get a lot of context on the business processes, and we're going to have to roll the dice. Now, does an executive want to roll the dice with budget? No. That sounds like R& D. That sounds really scary. But the fact of the matter is when AI projects are sold as if they're IT projects," Oh yeah, this result by this time," we end up with a big graveyard of pilots, which is what we've had for the last three years. Often we're not going to see a financial services firm until they've laid to rest millions of dollars on wasted and squandered projects with absolutely faulty misconceptions. And it is only then they will ask for market research and aim to ground their insights in some reasonable conception of what the hell is possible.
Jon Prial: So it's interesting, when I did my intro I was talking about trying to extrapolate software development to all product and services, and you've got to figure out what you're delivering against this market opportunity. And your view is that AI informs the company to look at the market differently. Is that a fair way to put it or not?
Daniel Faggella: I think AI can do a great many things. So within financial services, AI, low- hanging fruit. If you held a pistol against my temple, John, and you said," Dan, tell me, shortest path to money in banking." I would tell you two words, anomaly detection. That would be anti- money laundering, fraud, and cybersecurity. So, very quickly discerning patterns that will tell me where I am being screwed and where I may have the door open for serious compliance risk. So this is simply a pattern matching problem. However, that's not the gist of AI. If you have a five hour podcast I'll list them all for you, John. So, the fact of the matter is, yes, we can also look at search. We can search for documents and we can label them in terms of their various levels of legal risk, in terms of what we want to look at. Or find a 360 customer view of what they've done with us through various paperwork and contract search, for example. We can handle some low- hanging fruit customer service questions. There's a lot of reasons why that's hard in financial services, and I'd like to address that today. But we can do some of it, we can do some of it. We can also work on things like lending, so we can work on automating that process. We can work on calibrating that risk. So, can AI help us understand the market? Certainly it can. And in wealth management, actually there's a lot of very exciting applications of AI in that space. We've covered that space in great depth. But the fact of the matter is AI is a creeping and expanding set of capabilities. So our core work is called the AI Opportunity Landscape. This is essentially keeping tabs of all of the new verbs that are emerging. So a verb is something we can do. AI permits us to do a new set of things. And so that's always expanding, that's the tough part about AI?
Jon Prial: Is it harder do you think for the more established, larger companies, the big banks, and I don't want to say the word dinosaur, but sometimes I use the word dinosaur for the telcos and banks versus a young FinTech startup that provides the disruption? Because obviously that's a threat and maybe an acquisition opportunity. You're trying to take the blinders off and get the companies to look left and right and think about it. And you were going to mention a little more about knowing customers, a little bit of a know your customer kind of thing. How do you get them to recognize the threat as well as the opportunity to not do some of this work?
Daniel Faggella: Yeah, and that's a touchy line too, John, because our job as a market research firm is not to encourage people to buy and adopt AI. In fact, I've probably built my reputation on vehemently cutting down in merciless fashion what I consider to be garbage. And so that's a lot of my rap. So, I'm not interested in actually encouraging people to do AI for its own sake. However, I will tell you that we are going to need social proof as a motive to keep the dinosaurs chugging away. Now some" dinosaurs," some people might call Citibank a dinosaur, right? It's however old it is, a rather old company. Bank of America, rather old. Wells Fargo, for example, a rather old company. What they have as their Botox injection ability is they have the ability to not just buy pieces of companies, but to partner with basically whoever they want and even acquire potentially who they want. Now, is that enough to keep them alive? Can you Frankenstein modernity? Not necessarily, not necessarily. But you can do a decent job though. And so these big banks actually have a lot more action. When we go top to bottom, so if I were to tell you top seven US banks by rev, and I look at the top three, there's just a lot more interesting stuff going on in those top three than there are in the banks number 20 to 30 combined by a wide margin, simply because they have a number of factors. R& D budgets, some semblance of existing tech and venture investments and arms and wings that are focused on that kind of thing as well. They also have the repute, banks like Citi or JP Morgan has the repute to attract rather talented folks from the Carnegie Mellon's and the NYU's and the Stanfords of the world. So these are places that are ecosystems where AI can actually survive. You throw AI in some corner of Citizens Bank, a super regional here in the Northeast, who I respect, I used to bank with them, like them, they're going to have to pick their poison a little bit more selectively to figure out where they feel like they have the readiness. So the big folks, the dinosaurs with the factors I just told you about, I think are poised to be in a better spot, frankly, than the folks below them. But they're certainly not moving as fast as the fintechs, which you brought up as well.
Jon Prial: It's interesting because I'm thinking about, I don't know if it came from Fiserv or one of the other services provided to banks, but it used to be called pop money and [crosstalk 00:12: 06 ]. So I looked at these things and I realized they didn't invent this, but they went on and realized it was a necessary solution. We're not necessarily in the AI space. We're kind of in the how are they going to evolve? I use the word modernity, which I like, how are they going to become more modern? And what do they do? That is the challenge. And then AI could make it better for them if they think about it the right way. Is that fair?
Daniel Faggella: Yeah. If they think about it the right way. And again, there's a lot of shift that has to happen. So I would argue, and we do regularly, that yes, the tech has to improve. So for example, in chatbots, the core of natural language processing and conversational ability and machines needs to actually go up a couple of ticks for us to really see serious improvement. The very thought that Bank of America is going to have a better conversational agent than Google or Microsoft is so laughable that I won't even deign to laugh on your show about it. That's how laughable it is. So, I won't even deign to do that. Instead, I'll just say that the science itself isn't far enough in some of these areas. That's an issue. The vendor ecosystem is all rather nascent. That's also an issue. The biggest issue, bar none, is people adopting this stuff presuming they're doing push- button projects, presuming that, okay, well, this sounds neat. As opposed to understanding that there's a whole set of capabilities we need to build within our company, a whole way that we need to till our soil in terms of talent, culture, and resources. And that's the way we think about it. We have another article called Critical Capabilities, The Prerequisites to AI Deployment. The fact that that is completely neglected, and we're just thinking about outputs and capabilities without actually understanding what needs to be new and different in the business, is a big struggle. So executive grasp of what could I invest in and how is this setting us up to build the capabilities that will really let us be AI enabled? It's not about plug and play here. It is about tilling the soil in a new way. And there's ways to do that that are perfectly profitable. The fact of the matter is most of these pilot projects are done in the absolute opposite manner and we find ourselves with little silly sandbox projects after 750 grand. And we are where we are and there's not much to do about it. And then we get the phone call.
Jon Prial: I want to have a subtitle to that second article you referenced, called, don't run to the bright, shiny object, please.
Daniel Faggella: Yes, yes, yes, yes, yes.
Jon Prial: Be a little more thoughtful about your business.
Daniel Faggella: 100%, John.
Jon Prial: Let me generalize a bit. So do you see a different impact across different industries? Sometimes I'm afraid people think they could just draw a straight line, I have more data and therefore I have more AI opportunity. And I don't necessarily know that that's true. I think it's about the right data and the right solution against it. So do you agree with that and do you see subtleties across different industries?
Daniel Faggella: I do. There's a lot of really interesting subtleties. So we've got the public- facing articles at Emerj.com. Now, we have a lot more behind the wall, particularly the data and stats, which is what people ultimately pay for. But if people want to read our articles, literally thousands of articles between, let's say one and a half and 4, 000 words about everything from oil and gas, to construction, to retail. I've been doing this since like 2012. So there aren't that many people that have been interviewing AI industry oriented folks so far before it was cool. Nobody was putting venture money in it in 2013. I had three listeners a month. You know what I mean? It was insane. But at some point that became an advantage because people were like," Oh, geez, there's not that many people that have talked to these folks." So there are different trends in different sectors. For example, I'll give you a couple of high level things that are very fun to think about. So when we look at the retail space, there's an inordinate percentage of the AI market space that's focused on gaining revenue. So marketing, sales, user experience, it's actually genuine, not flim- flam, it's real in the retail/ e- commerce space. Now brick and mortar and e- commerce are different. But if I just lumped them together even, inordinately focused on revenue, there's a tremendous amount of the overall landscape focus in that dimension. There's a lot of ways we could break that out from personalization to recommendations, to messaging and email and SMS stuff, whatever. In the financial services domain, we see an inordinate focus on risk reduction, generally speaking, at least today. Not even efficiency, risk reduction. Not even efficiency, risk reduction. They're different. So we see, just even in terms of what kinds of ROI are being eked out, where the motives are we see a shift and that's a difference because of cultures of different sectors. So different sectors have different cultures. Also, the relative AI readiness and adoptability of AI in different sectors. So if we talk about retail, and we do include e- commerce in that mix, e- commerce companies are unbelievably advantaged because they're all rather young and they were built digital first. You can't be an e- commerce company with a pickup truck and a donkey or something. There's no Wells Fargo logo for an e- commerce company. This is no horse and buggy going on. So these are all modern companies that have a much greater likelihood of already having a very rife digital ecosystem, a talent base that's digital first and understands that. And data that's reasonably accessible compared to let's say, a bank in the Midwest that was founded 200 years ago and is still run by the great, great, great grandchild of whoever founded it in Omaha. And I have a lot of respect for those kind of companies, but it's a tougher ball game for adoption. So it has to do with culture, it has to do with adoptability. It also has to do with just what the markets are focused on. We see pharma is in a different place. They've done a lot of work in life sciences, a tremendous amount of work there. So yeah, it's neat to see the meta level shifts, particularly at a statistical level in terms of what the heck are people focused on?
Jon Prial: You get to the data but much later. So you do so much rich reporting. When you're getting called in which is the job title that calls you in? And then, I think more importantly, how do you go about getting company buy- in to your conclusions?
Daniel Faggella: Well, so okay. So these are two really robust questions that we've had a lot of meetings in- house about. So the first one's rather easy. By this point we've figured it out. Now, back in 2014 when I was doing some of my really early research work it was a little bit hodgepodge. Hardly anybody was really focused on AI. There were random odd balls that actually was people more on the tech side of the house that were calling us in to be the business voice on basically reality checking use cases and presenting libraries of use cases that they might use. Now, it's pretty consistently folks who are head of innovation, head of strategy. So it's leaders in innovation strategy. Now the reason that is, is because our research doesn't so much focus on... So the traditional Forrester Gartner model, which by the way, I respect tremendously. I think they're great companies. Some of my best advisors, people who are helping me grow this firm are ex- Forester. They spent 10 or 20 years there. My sales advisor spent 20 years at Forrester. A wonderful woman, extremely bright. But their model is generally focused on, hey, let's take these eight companies that do something similar. Let's say RPA in a certain industry, or let's say point of sale software, whatever it is. And let's compare them side by side in terms of features. The fact of the matter is AI is not really easily boxable in that same way right now. Most companies are asking the following question. They're asking," But what could I do with it?" And as it turns out showing that splay of capabilities is an entirely different kind of research, to show the things you don't know it can do that. That maybe your competitors are investing in that are damn well working. You want chatbots, but you know what? You actually don't. If you're 90% of banks, I'll either have very firm opinions about what you should be thinking about for conversational interfaces and I'll know exactly the vendor companies that are ever worth talking to. But I'm actually much more likely to, depending on your desired outcome, to open up the possibilities of other domains that maybe didn't get on your corporate radar, but are very important for you. So most people that come to us, innovation strategy. They're looking at, but where do we put it? But where do we allocate our money? But what's the best way to roll it forward to see an ROI. And these are folks that are thinking a little bit bigger picture, as opposed to just the head of fraud to say, compare the top six fraud vendors.
Jon Prial: Right. You want the dialogue much earlier. You're not worried about the spreadsheet that comes back from Forrester with a magic quadrant from Gartner. That's not where you are. You can't get there until you do the early thinking.
Daniel Faggella: That's exactly it. The other factor is there's not enough damn traction on the ground to really do a quadrant properly in most AI capabilities. So our job is to quadrant, in so much as we can, near term and long term value potential for vendors. But if Gardner's calling Oracle as one of their CRMs, they've got hundreds of customers to talk to. Enterprise size, big customers. They've got very concrete featureless. Brother, let me tell you this, John. This is a reality check for your listeners. Any AI company, show me somebody who's raised 30 or 50 million bucks. Show me their feature set six months from now after you take a screenshot of it today and tell me how the same it is. Go ahead, go ahead, go ahead. I update my AIOLs across eight different sectors every six months. And I can tell you, this is a shifting landscape and my job is to stay on top of this stuff. And so the fact of the matter is we're working on a little bit more quicksand. So yes, we can find vendors in the right features. I'm not saying it's impossible. I'm saying the splay of capabilities is quite open. And we tend to work with the folks who are asking," Hey, look, where is worth doubling down?" Even though things are moving, what are the tracks, the trends that are ultimately going to deliver value in our sector, that work for what our strengths are and where should we be headed? And that tends to be innovation and strategy leaders almost across the board in financial services.
Jon Prial: Innovation strategy. Now, can you do your work and not get called back again, or in six months they probably need to do a refresh with you and keep working through this?
Daniel Faggella: This will depend. So the core AI Opportunity Landscape Research is an annual subscription service, as many Forrester and Gartner services are. Again, we have a very different focus than the traditional market research firms, but the core services are recurring ones. That said, we will be brought on by a firm who's just looking for a particular vendor selection project for, let's say, churn reduction for a service. And they're really looking for a deep dive on vendors and a recommendation set, and some projection of capabilities and roadmap for the couple of years ahead, and some advisory along with that. And we may work with them for six or nine months. And to be frank, they just decide not to up on the full landscape there because it wasn't really in their purview. So sometimes it's going to be project level, but the core of our work for strategy and innovation folks is basically giving them that map, living and breathing, with advisory to go along with it and help them steer where to put their money to make sure they see a return.
Jon Prial: Excellent. So do a little quick shift here. So if an AI is in the middle of a solution now that a company is providing probably after spending some time with you, what about go- to- market? Do they need to modify what they do? Do end users care? Do they need to talk about we're protecting your data in this new world or buy it? What has to happen as they begin to take these AI solutions to market?
Daniel Faggella: Oh, man. Yeah. This is a great topic, John, and there's a couple of different opinions here that, for me, are really important to bring up. So we've done a lot of great interviews on this exact topic on the AI and business podcasts with folks from Oracle, to you name your startup in financial services. Really, really cool frames here. So I'll give you a few. Yes, when we are selling AI, I'll give you two categories of consideration. I consider these both to be ahas, and I think they'll be extremely meaningful for your listeners. The first set of ahas is that often for most, and when I say most, I mean something akin to 85, 90% of solutions, we are going to have to be serious about the integration deployment and time to ROI requirements of our solutions. If we are integrating with your data, Mr. Buyer, we were going to run into data harmonization issues, potentially, that are going to be kind of gunky. And I can't tell you exactly how long that's going to take. We have to be frank. We can't promise plug and play. We also, we're likely going to need some pretty consistent access to either your in- house IT, in- house data science or some in- house subject matter expert folks to help us train the system if it involves complex and unique training based off of your data. That's very different than just plugging in Salesforce. We're really going to need to experiment with you on our team. That may require actual folks that you pull out of their subject matter role and you keep paying them a salary to basically work with us, to make sure we get massive context on your problem. Whether it be fraud, cybersec, whatever, to make sure that the system can be trained hyper properly to the extremes of understanding the data and features of your unique business. So, when solutions are sold with no presumption that there is some science here... Remember, AI is not IT is the analogy. If the solution is being sold with none of that base reality baked in probably we're setting ourselves up to disturb the champion who we closed, and probably we're setting ourselves up for a little more than some money for a sandbox project, and potentially not really a great long- term relationship here. So that's one really important consideration.
Jon Prial: Interesting.
Daniel Faggella: So the second one is that what we are seeing, and this is over the last five years I've started to see a shift here. So something like two years ago we interviewed a company called EDITED. Their logo is the word edited, but in all caps. It's an e- commerce retail firm. They just raised another big round. I really like what they do. I talked to their CEO for quite a long time. They actually go into the way that they train their system and deliver their system. More and more solutions, John, are starting to go the route of actually not really trying to tie into, to even integrate very deeply with, or to even change at all the workflow of the end user. Windows, the same software of the workflows that people are already working with. Because as it turns out changing things in the enterprise, particularly for nascent applications that we can't necessarily tell if this is the wave of the future. It's one of a million things AI could do. It's very hard to get folks to commit to overhauling data infrastructure, to integrating a tremendous amount, to training off of proprietary data. So let me give you some good examples of companies that are making this easier to weave. So we interviewed a company called DroneDeploy. So DroneDeploy uses drones to look at the maintenance status of physical assets. So let's say telecom towers. So instead of having a guy manually climb a telecom tower every six months or one month or whatever it is, and look for rust on all these critical parts, we can have a drone potentially go up and down and be trained to highlight and select and color code, red, yellow, green, if you will, the status of those key parts. Now this company has decided that primarily the initial training element of taking all of that data, determining what red, yellow, green is, that they at DroneDeploy primarily are going to actually do that heavy lifting with the client early on, instead of having the client do it with their own data science. And then as soon as they can get that error very, very low, they're basically just going to treat it like it's a software. You go in and look at the same pictures that your guy climbing the ladder was going to show you. You're going to look at pictures except a drone is taking them and except these pictures have layered highlights featuring what the status is, or the presumed status is, of different pieces. So you can prioritize what images you look at and how quickly you move. So actually after some initial training and it does involve some, but the training, the technical training is all done by the company, not by the client. They're basically working with the same interface. So we're looking at minimizing workflow change. Let me give you another example. We'll talk about radiology. There's companies that are really trying to overhaul how radiology and diagnostics are being done. There's also companies, there's a firm called Aidoc, for example, we interviewed them some two years ago. They raised a tremendous amount of money since. Really, really interesting people. Started in Israel. They're expanding over here, as all Israeli companies do. The second office is always in New York if you're in Israel. Boringly predictable, but it makes sense, right? This is where the GDP is. Anyway, I like these guys a lot. They figured out certain kinds of radiology scans, lung cancer, being one primary example from them, where the radiologist sits in a bit of a pod, if you will, where they have images up in front of their face. And their job is to investigate these images and to determine and discern what should we do with these different patients? What should we recommend for them? What should we diagnose them with? What should we do for additional testing? Whatever. Now, what Aidoc does, at least purportedly, I'm never going to clean truth, I'm going to say what they claim, is that instead of actually changing any of that, what they're doing is they're piping the initial scan up into their secure cloud, if you will. They're layering it and labeling that with data. And by the way, the hospital never has to do that training. Aidoc has done that training off of various and sundry other data sets. And then they're just piping it into the same little hud environment that the radiologist is already looking at their images in. And now those images just have value laden right on top of them. So instead of having any piece of software really shift, all we're doing is we're creating a fork so that in addition to our own storage for these scans, these scans are also piping up to the cloud. And then X number of minutes later they're going into the hud in the same software the doc is already using, or the radiologist is already using, layering info. So to answer your question, when it is required we really do need to address the unique considerations of an AI solution, and be frank about that on the onset. But a lot of smart companies are asking the question, how can we use minimal datasets? How can we derive value from a tremendous amount of data that maybe we can get proprietary access to outside of the client, and simply layer data into their existing workflows in a way that's going to be worth a lot of money? But it's not going to actually involve data infrastructure overhauling and in- house data science talent, and all the things that make deployments very challenging sometimes. So we're seeing more and more companies move to the easy mode of AI value adding into existing workflows.
Jon Prial: Yeah, I like that, because we talked about the data you had, but it's the data that you need. We've often talked early days when we were doing applied analytics, before we even got to applied artificial intelligence. We've talked about derived data and we've heard the term data exhaust. All these things really do add that much more value to a solution, as opposed to just throwing everything together and seeing what comes out the other end. So again, I like the thoughtful approach to what do I need, how do I not impact the end user necessarily? Just make it better. That's really a nice piece.
Daniel Faggella: Yeah. And not all AI solutions can do that. But for the folks who can, I think, particularly in this COVID era where really hands- on, really lengthy, really complicated, initial integrations are just not going to be viable because of financial constraints, the companies that can nimbly integrate with fewer data sources, shake up fewer workflows, but add a targeted specific value that's worth something to the client. Frankly, I think in the next two years we are going to see a proliferation of AI firms that take that approach because we're realizing just how hard and gritty it is to be custom and white glove for every client.
Jon Prial: So just to wrap up, okay, AI is a tool, it's a different type of tool. When it's in the mix it's not the same as a piece of machinery or a piece of software. It's kind of in the mix. I like the fact that you mentioned early on that the banks struggle a little bit with governance inaudible matters. Talk to me about some of the elements of risk. You did a cool thing with a deep fake video at the UN a little bit. And I want to get you to wrap up a bit for me on how we make sure AI makes society better, which was a pretty cool TEDx talk that you had. So, let's talk a little bit about where all this is going and pitfalls that people should watch out for.
Daniel Faggella: Big time, yeah. Well, for me, John, this can go a couple of ways. So the long- term, my first TEDx talk about the long- term societal consequences of AI was 2014 about AI and neuro tech. I do think that in the coming 20 years we're going to see change so disruptive and violent to the human condition that for most people it'll borderline unfathomable. But that may not be the conversation for this talk. You're probably talking about more practical near term things. So in that regard, yes, we did a funny, deep fake presentation at United Nations headquarters. We took the head of UNICRI, which is the crime and justice wing of the UN and we made this woman say a bunch of things she never said. We actually had her talk about pineapple pizza of all things. So we just made her say a bunch of stuff and we kind of spooked the room. A room full of folks and delegates from around the world, and just to have them see the woman who's sitting in the room. I got to shake her hand and she laughed and she had a good time with it.
Jon Prial: Better than a punch in the nose I guess.
Daniel Faggella: Yeah, it's a little funny, right? It was like," Okay, what the heck is going on here?" There's a variety of categories of risk here. And I'll I'll address maybe two of them high level, because I know we only have so much time. One of them is the very real risk around unseen or borderline undetectable bias within business systems. Now, I am not an advocate that every AI solution is inherently evil and bias in some way. There are folks who default to that camp. I consider that a pretty repulsive stance. I do think that there are some instances where it is absolutely warranted and needs to be considered. We look at something like lending, where we are taking in demographic data, and there are hard compliance rules about what's not allowed to be in there. And by golly, we got to adhere to both the law and whatever values we want to stick to as a company. So some solutions that deal with some level of customer data will require additional scrutiny to ensure we're not crossing a regulatory threshold. In other words, the algorithm is proxying for something like zip code that might tie to race, let's say. There's areas where that gets a little spooky and a little risky. There's other areas like if we're doing a conversational interface, as a company are there certain kinds of things we're not going to let a bot handle and we're always going to do with a person? Or are there certain things that maybe we want to disclose about when it's a machine versus, whatever the case may be? These are value and interaction related. So there's that category. And the way that that works basically, is that smart executives and stakeholders consider those factors as they map out solutions. So when they decide, okay, I believe this AI application is going to be worthwhile, but it does involve this kind of customer data. We need to think from the beginning, how do we make sure we're crisply in line with the law and crisply in line with what we think the lived values of this company should be? And so long as that is asked in the room with smart data scientists and with smart subject matter experts, I believe a lot of the time the solutions are in that room. A lot of the time they are. Sometime maybe they're not, but I think a lot of the time they are. It's only a shame when those things aren't brought up when they need to be. But again, if you're searching for legal contracts or searching for invoice formatting or something, we don't have to worry too much about offending anybody here or breaking the law really. We just need to take in the OCR. So that's one category of insight. And I think that in- house band of folks who can think through the value and legal elements of AI, when it's warranted, when it's warranted, I think will be increasingly common as we start to see more and more cross- functionally AI teams in the enterprise. The second category here is more national security future of the human virtual experience here, where we are living in more and more digital ecosystems that are composed of whatever the algorithm bringith. And so, however those algorithms operate really are the string pullers of the emotive material, and content, and lessons learned, and angering things that are projected before our eyes. I was at Facebook headquarters in 2016 speaking with their head of core machine learning about six months before the election. And I talked to him off mic, of course, about the echo chamber idea that was starting to emerge. We were starting to see this. And I think now we see a lot of that. I think this also comes into play with, we could talk about China, for example. We have a pretty permeable digital ecosystem. China has the opposite. China does a really nice job of carefully molding the experience and the knowledge and the access to information of their citizenry. It's one approach to governing human beings. There's another approach that we have, but we've got a really nice permeable from the outside system. And so China can have valid Twitter accounts from their formal news stations just piping in propaganda. And they can also create oodles and oodles and oodles of accounts that can help to coax forth certain topics to become picked up in these algorithms to discern what displays something, what gets something hot, what gets a lot of anger rolling? And can really pipe that into the system. And so I think that the virtual ecosystems we're living in, in my personal opinion, I wish our own defense system here in the US was focused more on that than they were on things that rust. I think we should think about the cell phones in our pockets more than things that rust. So those are two big categories of risk that I've given a lot of presentations about for Interpol, the United Nations, etc.
Jon Prial: That's fantastic. There's so much to go. I'm fascinated but I think I still want some bridges to get fixed at the same time, but I'm with you.
Daniel Faggella: Yeah.
Jon Prial: I clearly understand your point though. There's a lot of places to go. We really are at the beginning of it. It's amazing how many years you've been doing this, and you mentioned you look six months later what software changes, two years later what your content's going to be, what your tech crosstalk talking about.
Daniel Faggella: It's crazy.
Jon Prial: We don't know. I think the important thing is that we keep ourselves thinking about the future, thinking broadly, thinking holistically. You made a couple of really good points throughout this interview about not digging in on the narrow stuff because I think that'll cause some problems. I think we need to stay broad. So Daniel Faggella, this was a fantastic conversation. Thanks for taking the time to be with us. It was a pleasure to chat and I look forward to seeing you again in writing in and wherever you show up.
Daniel Faggella: You bet, my man. Hey, thanks so much for having me here, John.
DESCRIPTION
With the growing pervasiveness of AI and machine learning, knowing how to effectively implement it in software development is key. But the idea of “just take X and add AI” isn’t a cure-all. So, when? How? Where?
Daniel Faggella is our guest on this episode of the Georgian Impact Podcast. He is the CEO and Head of Research at Emerj Artificial Intelligence Research, a research and advisory company working with business leaders to help develop a winning strategy.
You’ll Hear About:
● The difference between AI and IT, and how this impacts where a CEO may want to invest.
● How big banks can make better use of AI as they embrace modernity.
● How to build the capabilities to leverage AI within your company.
● How to take an AI solution to market?
Who is Daniel Faggella?
Founder and Head of Research at Emerj Artificial Intelligence Research, Daniel is an internationally recognized speaker on the use cases and ROI of A.I. in business. Regularly called upon by global enterprises in financial services and security, Daniel has spoken for many of the largest and most reputable organizations including the World Bank, the United Nations, INTERPOL, and more.
Today's Host
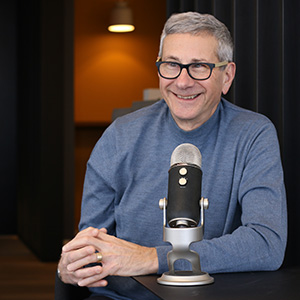
Jon Prial
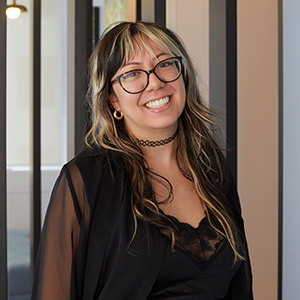
Jessica Galang
Today's Guests
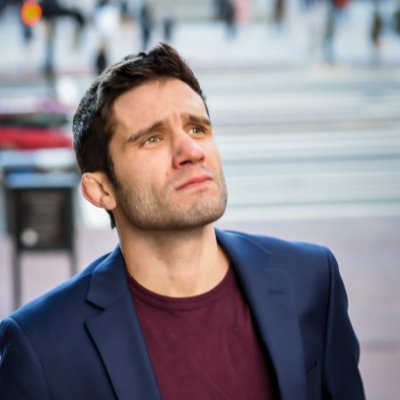